线性函数预测
import tensorflow as tf
import numpy as np
import matplotlib.pyplot as plt
x_data = np.random.rand(100).astype(np.float32)
y_data = x_data*0.1 + 0.3
Weights = tf.Variable(tf.random_uniform([1], -1.0, 1.0))
biases = tf.Variable(tf.zeros([1]))
y = Weights*x_data + biases
# biases_stop = tf.stop_gradient(biases)
# y_stop = Weights*x_data + biases_stop
# loss_stop = tf.reduce_mean(tf.square(y_stop-y_data))
loss = tf.reduce_mean(tf.square(y-y_data))
optimizer = tf.train.GradientDescentOptimizer(0.1)
train = optimizer.minimize(loss)
# train_stop = optimizer.minimize(loss_stop)
init = tf.global_variables_initializer()
sess = tf.Session()
sess.run(init)
for step in range(1001):
sess.run(train)
# if step < 10:
# sess.run(train)
# else :
# sess.run(train_stop)
# if sess.run(loss_stop) > 0.05:
# sess.run(train)
if step % 20 == 0:
print(step, sess.run(Weights), sess.run(biases), sess.run(loss))
# draw
Weights = sess.run(Weights)
biases = sess.run(biases)
y = sess.run(y)
# draw
plt.plot(x_data, y_data, "+")
plt.plot(x_data, y)
plt.show()
# gradients = optimizer.compute_gradients(loss)
# print(sess.run(gradients))
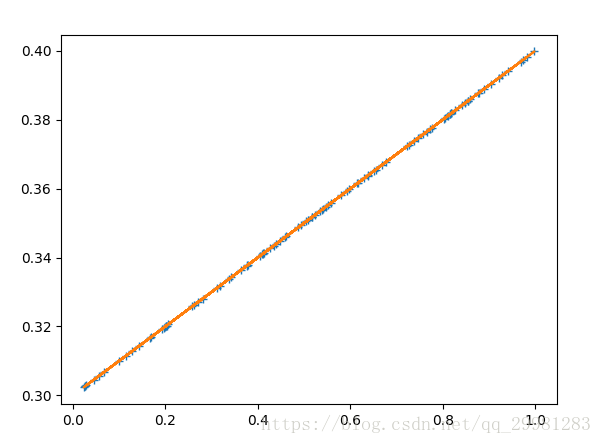
# 可以停止后向传播,只进行前向传递
tf.stop_gradient()
# 能够返回运算过程的梯度 return (gradients, variables)
gradients = optimizer.compute_gradients(loss)