AWN
2020-CVPRw-Any-Width Networks
来源:ChenBong 博客园
- Institute:University of North Carolina at Chapel Hill
- Author:Thanh Vu, Marc Eder, True Price, Jan-Michael Frahm (H 54)
- GitHub:https://github.com/thanhmvu/awn
- Citation:/
Introduction

Motivation
slimmable network工作中存在的问题: 每个宽度的网络都需要各自的bn统计量, 之前的2个解决方法:
- 在训练过程中就设置私有bn (SNet, 增加存储开销, 在宽度个数不多的情况下不明显, 在宽度个数多的时候e.g. 20 widths on MB V2, 增加18.5%的model size)
- 训练后的bn校正 (USNet, MutualNet 等其他one-shot nas的标配做法; 需要post process的过程)
针对这2个方案存在的问题, 提出了一种下三角卷积层, 替换常规卷积层, 实现不增加存储开销, 无需post process, 训练完毕后可以在任意宽度进行推理
Contribution
Method
不同宽度的bn层需要重新校正的原因是什么?
以MLP为例
(oldsymbol{y}_{i}^{left{m_{i} ight}}=oldsymbol{W}_{i}^{left{m_{i} imes m_{i-1} ight}} oldsymbol{x}_{i}^{left{m_{i-1} ight}})
第 (i) 层的全连接层神经元个数是 (m_i) , 上一层全连接层神经元个数是 (m_{i-1})
当乘上宽度系数 (alpha) 后, 该层的神经元个数变为 (k_{i}=alpha m_{i})
例如, k=1时:
(egin{aligned} oldsymbol{y}^{{1}} &=oldsymbol{W}^{{1}} oldsymbol{x}^{{1}} \left[y_{1} ight] &=left[w_{11} ight]left[x_{1} ight] \ &=left[w_{11} x_{1} ight] end{aligned})
k=2时:
(egin{aligned} oldsymbol{y}^{{2}} &=oldsymbol{W}^{{2}} oldsymbol{x}^{{2}} \left[egin{array}{l}y_{1} \ y_{2}end{array} ight] &=left[egin{array}{ll}w_{11} & w_{12} \ w_{21} & w_{22}end{array} ight]left[egin{array}{l}x_{1} \ x_{2}end{array} ight] \ &=left[egin{array}{l}w_{11} x_{1}+w_{12} x_{2} \ w_{21} x_{1}+w_{22} x_{2}end{array} ight] end{aligned})
使用bn层后, 全连接层的输出 (mathcal y) 会按照累积的统计量 mean, var进行 normalization后 再作为下一层的输入
以只有一个样本为例, 对于不同宽度的全连接层:
(y_{1}=left{egin{array}{ll}w_{11} x_{1} & ext { if } k=1 \ w_{11} x_{1}+w_{12} x_{2} & ext { if } k=2 \ ldots & ldotsend{array} ight.)
(egin{aligned} mathbb{E}left[y_{1}^{{1}} ight] &=mathbb{E}left[w_{11} x_{1} ight]=mu_{1}^{{1}} \ mathbb{E}left[y_{1}^{{2}} ight] &=mathbb{E}left[w_{11} x_{1}+w_{12} x_{2} ight] \ &=mathbb{E}left[w_{11} x_{1} ight]+mathbb{E}left[w_{12} x_{2} ight] \ &=mu_{1}^{{1}}+mathbb{E}left[w_{12} x_{2} ight] end{aligned})
除非 (mathbb{E}left[w_{12} x_{2} ight]=0) , (y^{{1}}) 和 (y^{{2}}) 才能服从相同的分布
即简单地设 (W_{ij}=0) 当 (i<j) , 得到 triangular convolution/fc layer
用 triangular convolution layer 替换原始的conv/fc layer的网络称为 AWNs

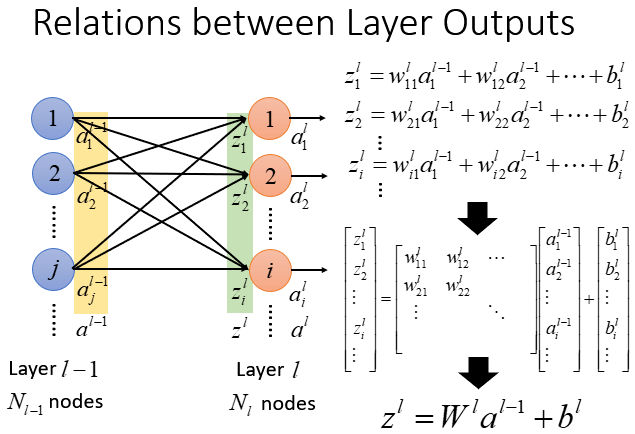
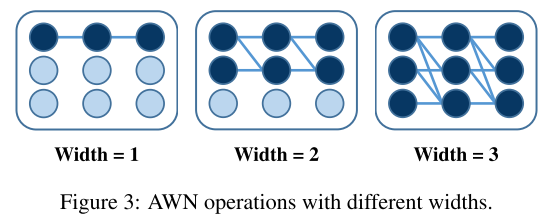
对于AWNs, 有2种训练方式:
- AWN: k-width training (和 S-Net 相同)
- AWN+RS: random sample training (和US-Net相同)
第 (i) 层的全连接层的神经元个数是 $$
Experiments
LeNet-3C1L on MNIST
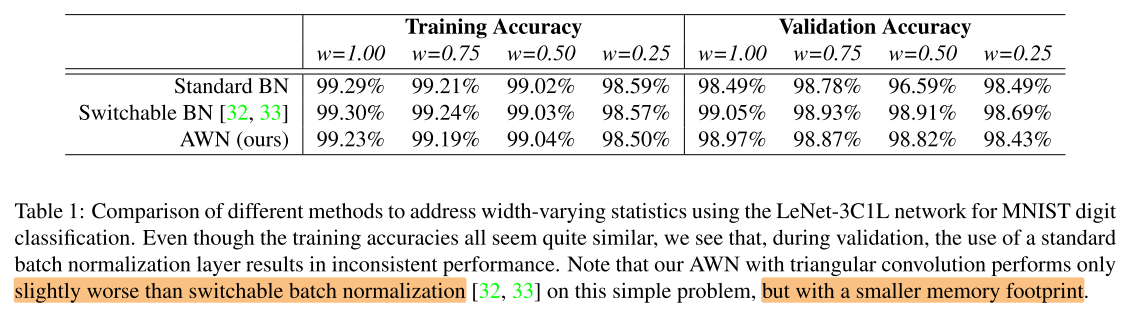
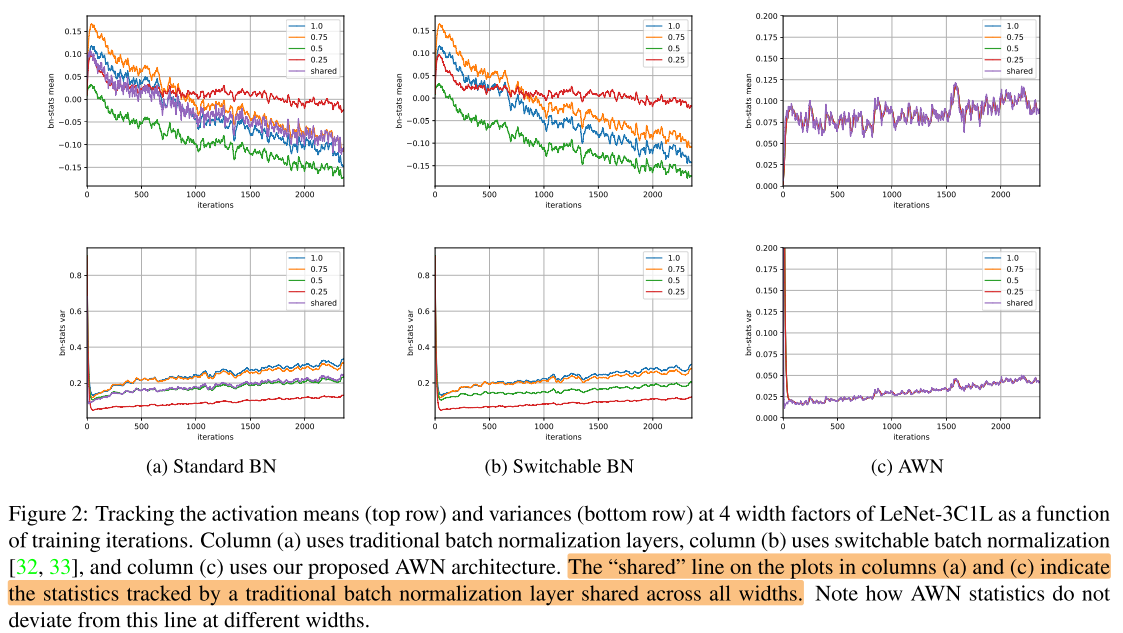
setup
由于AWNs会减少接近一半的参数量, 一次训练中AWNs使用的网络宽度扩大了 (sqrt 2) 倍, 以保持参数量和原网络一致
- SGD, momentum=0.9, batch_size=128
- LeNet-C31L
- S-Net, US-Net, AWN, AWN+RS
- lr: 0.01 (decay by 0.1 at 50%, 75% epochs)
- FashionMNIST
- 20 epochs
- weight_decay=0.0
- CIFAR-10/CIFAR-100
- 100 epochs
- weight_decay=5e-4
- MobileNetV2
- S-Net, US-Net
- CIFAR-10/CIFAR-100
- 100 epochs
- weight_decay=5e-4
- lr=0.1 (decaying linearly)
- CIFAR-10/CIFAR-100
- AWN
- CIFAR-10
- 700 epochs
- weight_decay=5e-4
- lr=0.02 (decay by 0.2 at 500, 600 epochs)
- CIFAR-100
- 100 epochs
- weight_decay=5e-4
- lr=0.1 (?)
- CIFAR-10
- AWN+RS
- CIFAR-10
- 350 epochs
- weight_decay=?
- lr=0.01 (decay by 0.1 at 250, 300 epochs)
- CIFAR-100
- 1050 epochs
- weight_decay=1e-3
- lr=0.01 (decay by 0.1 at 750, 900 epochs)
- CIFAR-10
- S-Net, US-Net
main results
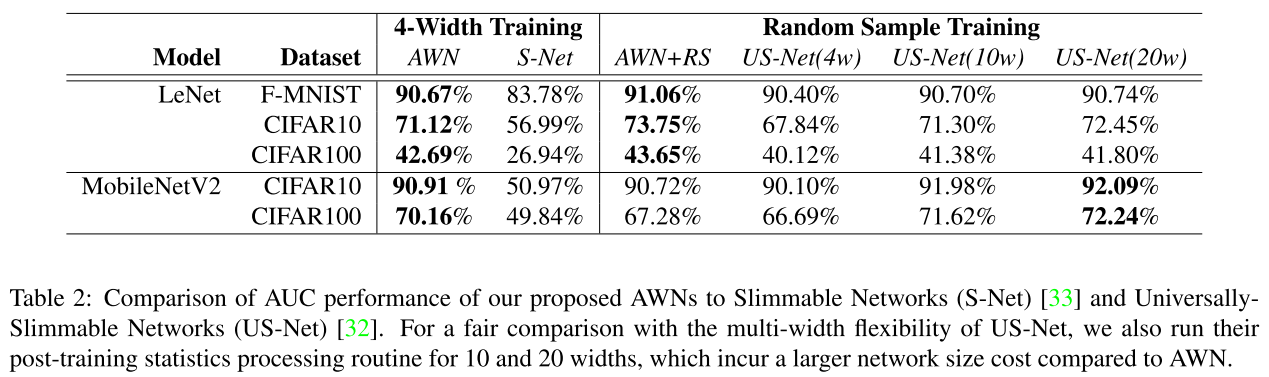
Area Under the Curve(AUC)

Conclusion
Summary
pros:
- 提出了一种天然支持不同宽度, 不改变bn统计量的卷积/全连接层, 下三角权重矩阵
cons:
- 扩大了网络的宽度, 一定程度上是不公平的比较
- MobileNetV2上的实验设置很复杂, 有的epoch数特别大, 其他参数像是精心选择过, 且代码只开源了 lenet cifar10
- 估计在大数据集上效果不佳
Question:
- 使用下三角的conv/fc替代原始conv/fc后, 参数量计算量都减半? 宽度扩大为原来的 (sqrt 2) 倍, 参数量, 计算量都恢复到和原网络一致?